Time Series Analysis Services & Solutions
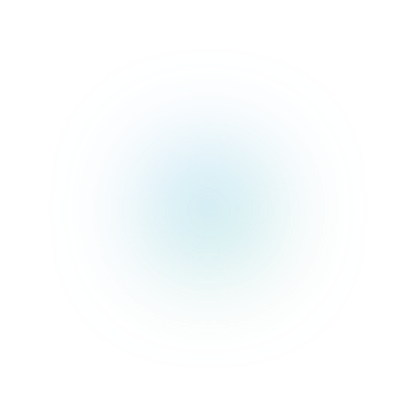
What is Time Series Analysis?
A time series is a logically ordered collection of data points that have been collected over a period of time. A time series is distinguished from other categories of data sets by its primary characteristic of being indexed or enumerated in time order. If you were to depict the nodes of time series data on a graph, one of the axes would always be time.
Moreover, A time series metric is a type of data that is monitored at a specific interval of time. For example, a metric could indicate the quantity of inventory that was sold in a store from one day to the next.
Time series data is ubiquitous, as time is a component of all observable phenomena. Sensors and systems are perpetually emitting an endless stream of time series data as our world becomes more instrumented.
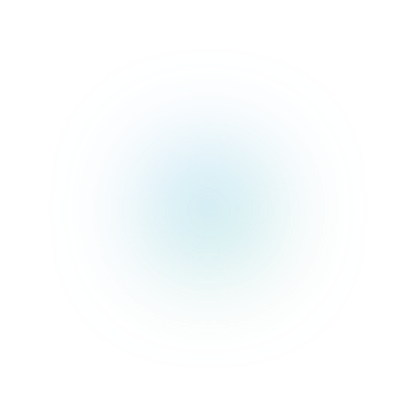
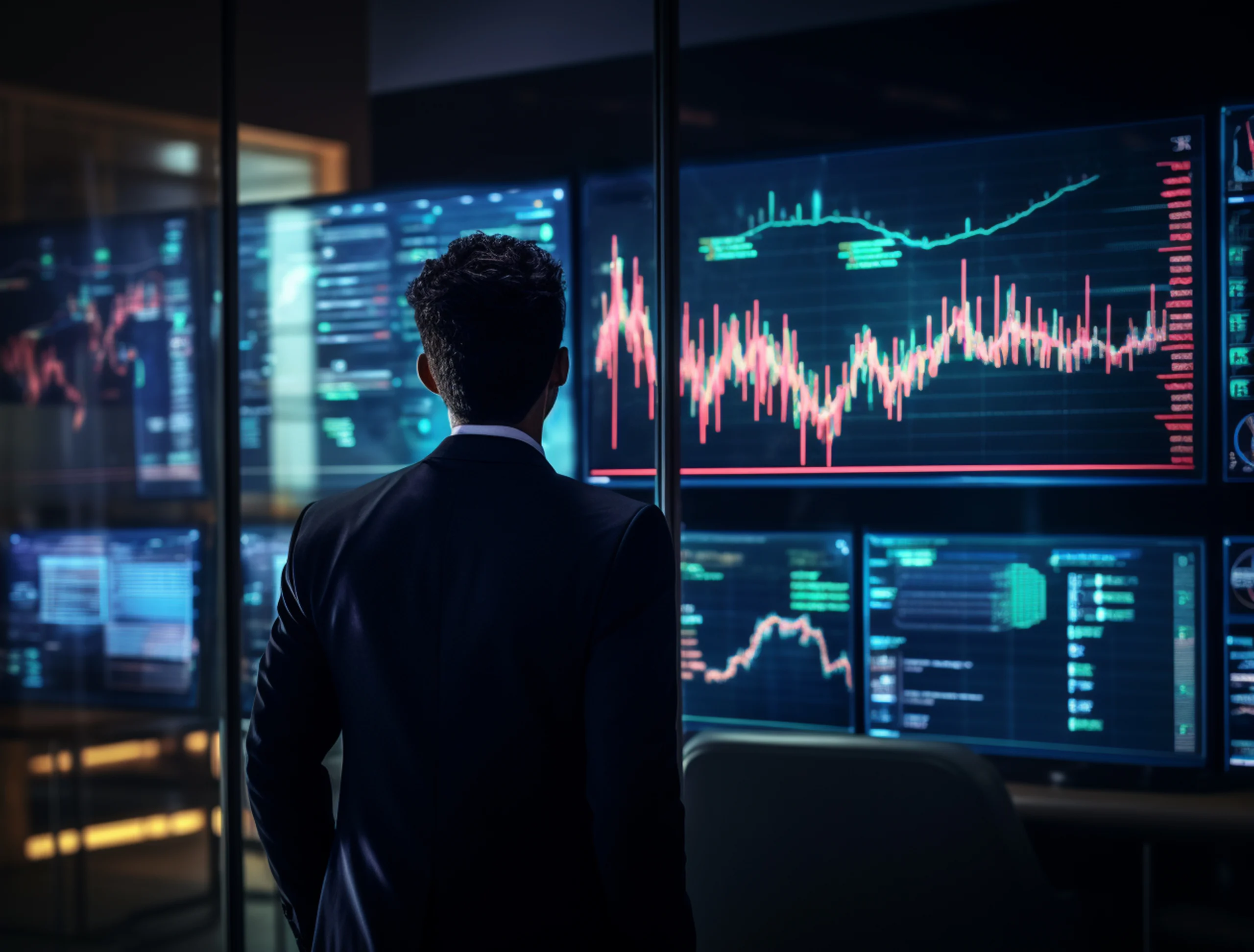
Why organizations use time series data analysis?
Time series analysis is employed to evaluate non-stationary data, which is data that is subject to time-dependent fluctuations. Time series analysis is frequently employed in industries such as finance, retail, and economics due to the constant fluctuations in currency and sales. Moreover, Automated trading algorithms are particularly effective in demonstrating the application of time series analysis in stock market analysis. Similarly, time series analysis is an excellent tool for predicting weather changes, enabling meteorologists to anticipate a wide range of events, including future years of climate change and tomorrow’s weather report. Here are some examples of time series analysis in action:
1. Data regarding the weather
2. Measurements of precipitation
3. Temperature measurements
4. EKG monitoring of heart rate
5. Electroencephalography (EEG)
6. Sales conducted on a quarterly basis
7. Values of stocks
8. Stock trading that is automated
9. Industry predictions
10. Interest rates
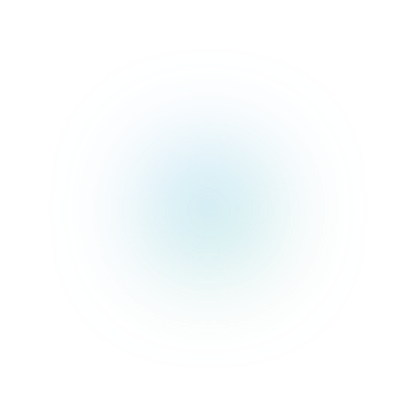
Time Series Analysis Types
Complex models are occasionally necessary for analysts to create, as time series analysis encompasses numerous categories or variations of data. Nevertheless, analysts are unable to generalize a specific model to every sample and account for all variances. However, A lack of fit can result from models that are overly complex or attempt to perform too many tasks. The analysis is biased and the forecasts are inaccurate as a result of the models’ inability to differentiate between random error and genuine relationships due to a lack of fit or overfitting. Time series analysis models includes.
Time series analysis models includes
Classification
Identifies and assigns categories to the data.
Curve fitting
Plots the data along a curve to study the relationships of variables within the data.
Descriptive analysis
Identifies patterns in time series data, like trends, cycles, or seasonal variation.
Explanative analysis
Attempts to understand the data and the relationships within it, as well as cause and effect.
Exploratory analysis
Highlights the main characteristics of the time series data, usually in a visual format.
Forecasting
Predicts future data. This type is based on historical trends. It uses the historical data as a model for future data, predicting scenarios that could happen along future plot points.
Intervention analysis
Studies how an event can change the data.
Segmentation
Splits the data into segments to show the underlying properties of the source information.
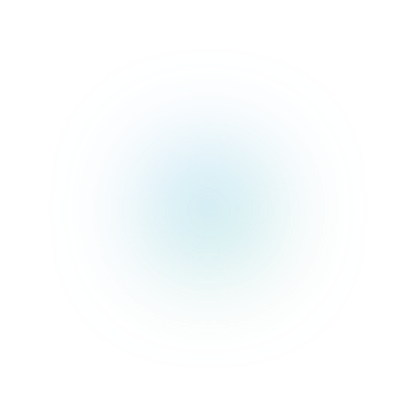
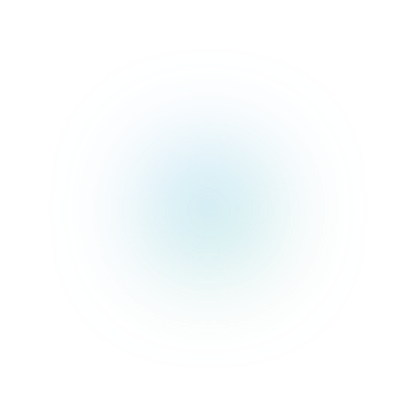
Importance of time series analysis
The capacity to manage time series data has become increasingly important as more connected devices are implemented and data is anticipated to be collected and processed in real-time. However. this will become more critical in the coming years as the Internet of Things, AI, and devices continue to play a more significant role in all of our lives.
At its center, the Internet of Things is a term that denotes a network of literally billions of connected devices that are constantly exchanging and generating data. This transition has already begun in “smart” residences across America in a personal context. Moreover, Your thermostat is aware that it must lower the blinds in a room to assist in temperature regulation when it reaches a specific temperature. Alternatively, your smart home gateway is aware that all doors must be locked and all lighting must be turned off immediately upon the final individual departing the residence. Yet, it would not have been possible to reach this stage without an interconnected network of sensors that exchange information continuously, which underscores the importance of time series analysis.

Classification of data in Time Seires
Stock time series data means measuring attributes at a certain point in time, like a static snapshot of the information as it was.
Flow time series data means measuring the activity of the attributes over a certain period, which is generally part of the total whole and makes up a portion of the results.

Data variations
Variations may occur periodically in time series data: Functional analysis can identify significant events by identifying the patterns and relationships in the data. Trend analysis is the process of identifying a consistent trend in a specific direction. There are two categories of trends: stochastic, which is random and unfathomable, and deterministic, which can be identified by identifying the underlying cause.

What is a time series graph?
Time series graphs are essentially representations of time series data on one axis (usually Y) against time on the other axis (usually X). A more intuitive and accessible method of illustrating trends or patterns is often achieved through the use of time series data points in graphs.

What are time plot statistics?
Time series forecasting uses information regarding historical values and associated patterns to predict future activity. These forecasting methods include; Cyclical Fluctuation Analysis, Seasonal Pattern Analysis & Trend Analysis. Success is not guaranteed, as is the case with all forecasting techniques. This is a frequent application of machine learning. Error, Trend, Seasonality Forecast (ETS), Autoregressive Integrated Moving Average (ARIMA), and Holt-Winters are its classical predecessors. Working with time-based data (years, days, hours, minutes) to extract latent insights that inform decision-making, time series modelling (a forecasting method based on time series data) enables individuals to “see things” ahead of time. Time series models are highly beneficial when dealing with serially correlated data. The majority of businesses utilize time series data to evaluate competitive positioning, website traffic, sales projections for the upcoming year, and other factors. Learn more about time series forecasting methods, such as, smoothing-based models, and models that incorporate seasonality.

How we can understood and used time series data?
Time series data is collected, preserved, visualized and analyzed for a variety of purposes in a variety of domains: Time series analysis is employed in data mining, pattern recognition, and machine learning for the purposes of clustering, classification, anomaly detection, forecasting, and querying by content. Signal detection and estimation are achieved through the utilization of time series data in signal processing, control engineering, and communication engineering. Time series analysis is employed in the fields of econometrics, quantitative finance, seismology, meteorology, and geophysics to forecast.

Identifying time series data
Time series data is distinctive in that it is arranged in a natural time sequence, which is determined by the order in which it was observed. The primary distinction between time series data and regular data is that it is subject to ongoing inquiry. To ascertain whether the dataset in question is a time series, it is frequently straightforward to observe whether one of the axes is time-based.

Time series data
A collection of observations (behaviours) for a single subject (entity) at various time intervals (generally equally spaced as in the case of metrics, or unequally spaced as in the case of events) is referred to as time series data.. For instance, the maximum temperature, humidity, and wind (all three behaviours) in New York City (a singular entity) were collected on the first day of each year (multiple intervals of time). Time series data is distinguished from other categories of data by the significance of time as an axis.

Where is time series data stored?
Massive volumes of time series data are frequently ingested, necessitating a database that is specifically engineered to accommodate its scope. Summarization, data lifecycle management, and large-scale scanning of numerous recordings are the distinguishing characteristics of time series data that distinguish it from other data workloads. This is the reason why it is recommended to store time series data in a time series database that is designed to handle metrics, events, or measurements that are time-stamped.
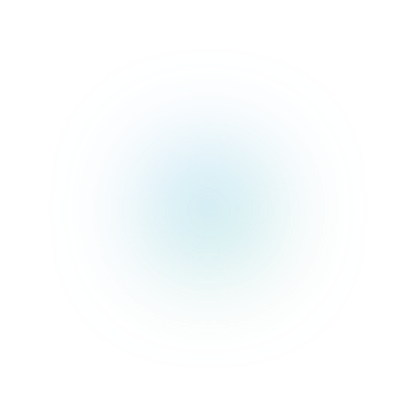
Rationale & Benefits for Choosing AI Culture to Develop Predictive Analytics Solutions
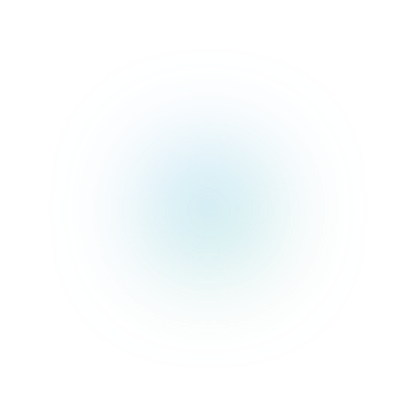
We are a Predictive Analytics Company Which Enables You to Dive into Data-Driven Insights

Hanna Sajira
Benefits of Our Time Series Analysis Services

Accurate Forecasting
Gain insights into future trends and patterns, enabling proactive decision-making and strategic planning.

Operational Efficiency
Optimize resource allocation, inventory management, and production schedules based on predictive analytics.

Risk Mitigation
Identify potential risks and vulnerabilities, allowing preemptive measures to mitigate adverse impacts.

Enhanced Performance
Improve business performance metrics such as revenue, profitability, and customer satisfaction through data-driven insights.

Competitive Advantage
Stay ahead of the competition by leveraging advanced analytics to anticipate market changes and capitalize on emerging opportunities.
Why Work With US?

A Highly Professional Team
In all that we do for our customers, we aim to achieve excellence.

Scalable Results
Our focus is on the long term. Our consulting services and solutions for predictive analytics may expand to meet your company's needs as it grows.
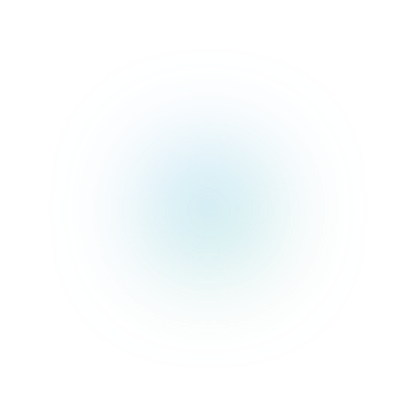
Get Started With Us Today

Contact Us
To get in touch with us, fill out the NDA-protected contact form, book a time a Zoom meeting with our experts.

Get a Consultation
Connect with us on a call with our team to know the feasibility of your project idea.
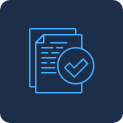
Cost Estimate
Based on the project requirements, AI Culture team share a project proposal with budget and timeline estimates.

Project Completion
Once the project is signed, we bring together a team from a range of disciplines to kick start your project.
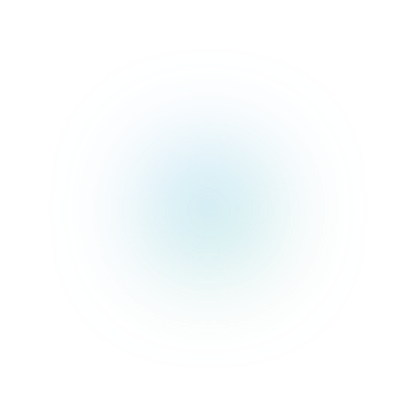
Frequently Asked Questions (FAQs)
What is Predictive Analysis?
How can Predictive Analysis Benefit My Business?
Predictive analysis offers numerous benefits for businesses across various industries. It optimize resources allocation to improve operational efficiency, mitigates risks by identifying potential threats, promotes revenue growth through personalized marketing and customer retention strategies, and enhances decision-making by providing actionable insights.
What Types of Data are Used in Predictive Analysis?
Diverse data sources, including structured data (e.g., sales transactions, customer demographics, and inventory levels), unstructured data (e.g., social media feeds, customer reviews, and text documents), and semi-structured data (e.g., XML files and JSON data), are analyzed in predictive analysis.
How Accurate are Predictive Analysis Models?
The accuracy of predictive analysis models depends on various factors, including the quality of data, the complexity of algorithms, and the domain expertise of analysts. While predictive models can provide valuable insights and forecasts, it’s essential to continuously validate and refine them to improve accuracy and reliability over time.
Is Predictive Analysis Suitable for Small Businesses?
Certainly, predictive analysis is advantageous for businesses of all sizes, including small and medium enterprises (SMEs). Predictive analysis can be employed by SMEs to optimise operations, obtain insights, and compete effectively in their respective markets, thanks to the availability of user-friendly tools and platforms and technological advancements. Nevertheless, it is essential to customise predictive analysis solutions to the unique requirements and assets of small businesses in order to guarantee scalability and cost-effectiveness.
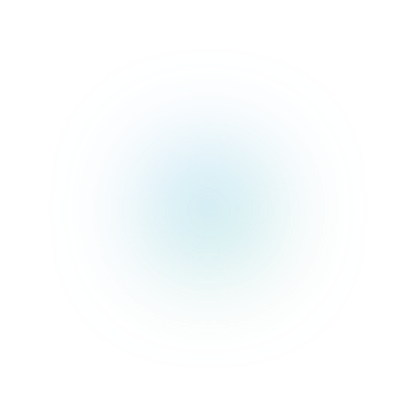
Contact Us
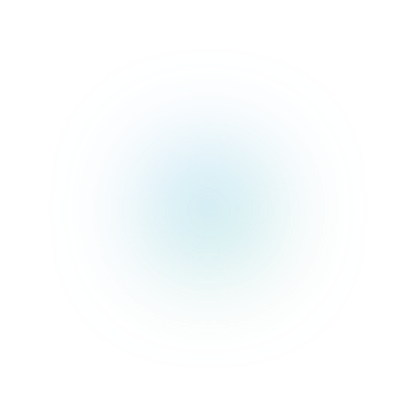
Reach out for tailored solutions & expert support. Let’s drive your business forward.