With rapid progress in AI over the last few years, we’ve seen powerful models such as OpenAI’s ChatGPT, Google Bard, and Meta’s Llama leading the pack. However, the next phase of AI entails reasoning-first models capable of providing outputs with a detailed explanation to their reasoning, as well as getting progressively smarter with each interaction just like as we are seeing DeepSeek R1 is the future of AI reasoning.
This vision is now a reality with DeepSeek R1 is the future of AI reasoning and pioneering large language model (LLMs) that is set to transform our interactions with AI technology.
In this blog, we will cover the following topics:
- An in depth look at what DeepSeek R1 is alongside how it stands out from traditional AI models.
- Advanced features that enable it to perform reasoning.
- Industries where DeepSeek R1 has the most valuable applications.
- Tips and guidelines on how to use and prompt engineer DeepSeek R1.
- Reasoning enabled AI models like DeepSeek R1 deepen typically predict and impact the future.
You should know 👉🏽8 Free Courses to Master Large Language Models
What is DeepSeek R1?
DeepSeek R1 is the future of AI reasoning and is a unique breakthrough in open-source language models developed by a Chinese AI research team that places emphasis on DeepSeek R1’s reasoning functionality. Unlike traditional AI that depends on recalling information, DeepSeek R1 was made to emphasize logic-based problem solving, critical thinking, and the ability to perform high level decisions.
DeepSeek R1 is The Future of AI Reasoning
Most AI models excel at predicting text but lack the ability to think through problems step by step. DeepSeek R1 is built as a “reasoning model”, setting new standards in AI by:
- Outperforming existing models in reasoning tasks like mathematics, coding, and scientific problem-solving.
- Democratizing AI development through its open-source accessibility.
- Enhancing model efficiency while reducing computational overhead.
It (DeepSeek R1) competes directly with OpenAI’s GPT-4, Claude 3.5, and Google’s Gemini models, proving that reasoning-based AI will drive key advancements in large model progress in 2025 and beyond.
DeepSeek R1 vs. Other AI Models
Feature |
DeepSeek R1 |
GPT-4 | Claude 3.5 |
Gemini 1.5 |
Reasoning-Based AI |
✅ |
⚠️ Limited | Yes✅ | ⚠️ Limited |
Open-Source |
Yes ✅ |
❌ | No ❌ |
❌ |
Chain of Thought (CoT) | ✅ Advanced | Basic ✅ | Advanced |
✅ Basic |
Reinforcement Learning |
Yes ✅ |
✅ | Yes |
✅ |
Computational Efficiency | ✅ Optimized | ❌ High | ✅ Optimized |
❌ High |
Runs Locally |
✅ Yes (14B Model) |
❌ No | No | ❌ No |
Understanding DeepSeek R1’s Core Architecture
Unlike traditional AI models that prioritize pattern recognition, DeepSeek R1 is designed for thoughtful problem-solving.
-
Neural Network with 671 billion Parameters
At the heart of DeepSeek R1 is an impressive neural network comprising 671 billion parameters. While size matters, what truly sets DeepSeek R1 apart is its innovative training techniques:
- Chain of Thought (CoT) prompting – enables step-by-step reasoning.
- Reinforcement learning – helps the AI model improve based on previous mistakes.
- Model distillation – allows smaller models to inherit the knowledge of the full-scale model, making AI more accessible.
These AI training methodologies improve accuracy, adaptability, and problem-solving skills, making DeepSeek R1 one of the most intelligent and self-improving LLMs available.
Breakthrough Innovations of DeepSeek R1
-
Chain of Thought (CoT) Reasoning: AI That Thinks Out Loud
Traditional AI models predict the next word based on probabilities, often generating fluent but inaccurate responses. DeepSeek R1 employs Chain of Thought (CoT) reasoning, which forces the AI to explain its thought process step by step before arriving at an answer.
Example: If asked, “What is 14 × 19?”, a typical AI might guess a number, while DeepSeek R1 would show each multiplication step before providing the answer.
-
Reinforcement Learning: Teaching AI to Learn from Experience
Unlike traditional training methods, which involve feeding models vast amounts of text, DeepSeek R1 applies reinforcement learning—where the AI actively evaluates its own responses and improves.
How it works
- Group Relative Policy Optimization: Instead of being given the correct answer, DeepSeek R1 compares previous and new answers, scores its performance, and self-adjusts over time.
- Human-Like Learning Process: Just like humans learn from trial and error, DeepSeek R1 refines its understanding to achieve more accurate, nuanced responses.
-
Model Distillation: Making AI More Accessible
A 671-billion-parameter model is powerful but requires significant computational resources. To make AI more accessible, DeepSeek R1 uses model distillation:
- A large model (“teacher”) trains a smaller model (“student”).
- The distilled models retain most of the teacher’s capabilities while requiring less computing power.
- Smaller versions can run on home devices with just 48GB of RAM, making advanced AI available to a wider audience.
-
Open-Sourcing of Reasoning Tokens
Most AI companies guard their training processes as trade secrets. DeepSeek R1 is different it open-sources its reasoning tokens, allowing:
- Developers to analyze the model’s thought process.
- Researchers to explore AI interpretability and bias reduction.
- A broader community to contribute improvements.
By openly sharing training insights, DeepSeek R1 is setting a new transparency standard in AI research.
DeepSeek R1’s Core Innovations
Innovation | How It Works | Why It’s Important |
Chain of Thought (CoT) Reasoning | AI breaks problems into logical steps before answering. | Improves accuracy and transparency in AI responses. |
Reinforcement Learning | AI evaluates past responses and improves future ones. | Learns from mistakes and refines decision-making. |
Model Distillation | Smaller models inherit knowledge from a large model. | Reduces computational needs while keeping performance high. |
Open-Sourcing Reasoning Tokens | Developers can access AI’s reasoning process. | Increases transparency and enables AI research improvements. |
Real-World Applications of DeepSeek R1
DeepSeek R1’s reasoning capabilities make it invaluable across multiple industries:
1. AI-Assisted Coding
- Generates and debugs complex code.
- Helps developers write optimized programs faster.
- Supports multiple programming languages.
2. Healthcare & Medical Research
- Provides AI-driven diagnostics.
- Assists in medical literature analysis.
- Enhances clinical decision-making with logical reasoning.
3. Education & Personalized Learning
- Creates custom learning experiences for students.
- Helps teachers generate AI-powered lesson plans.
- Tutors’ students in math, science, and programming.
4. Supply Chain & Logistics
- Optimizes multi-step logistics processes.
- Improves demand forecasting.
- Reduces delivery delays with AI-generated planning.
DeepSeek R1 Applications Across Industries
Industry | How DeepSeek R1 Helps |
AI-Assisted Coding | Generates and debugs complex code, improves efficiency. |
Healthcare & Medical Research | AI-powered diagnostics, medical literature analysis. |
Education & Personalized Learning | Creates lesson plans, AI tutoring, student assessments. |
Finance & Trading | Detects fraudulent transactions, AI-driven market analysis. |
Supply Chain & Logistics | Optimizes deliveries, enhances demand forecasting. |
Best Practices for Using DeepSeek R1
To maximize DeepSeek R1’s reasoning capabilities, follow these prompting techniques:
1. Use simple, direct prompts
- ✅ “Explain how photosynthesis works step by step.”
- ❌ “Write me a complex paper on photosynthesis.”
2. Employ “few-shot” prompting
- ✅ Provide 1-2 examples to guide AI responses.
3. Encourage extended reasoning
- ✅ “Think carefully and explain your thought process.”
Running DeepSeek R1 Locally
To test DeepSeek R1 on your own machine, follow these steps:
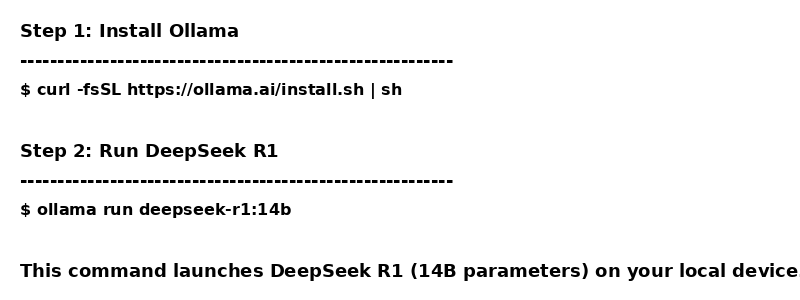
Technical Requirements to Run DeepSeek R1 Locally
Requirement | Minimum Specs | Recommended Specs |
RAM | 24GB | 48GB+ |
GPU | NVIDIA RTX 3090 | NVIDIA A100 |
Storage | 100GB | 500GB SSD+ |
OS | Linux/macOS/Windows | Linux/macOS |
Software | Ollama, Python 3.10+ | Ollama, CUDA for GPU |
Future of AI: What’s Next for DeepSeek R1?
DeepSeek R1 is paving the way for reasoning-first AI models, moving from data-intensive pre-training toward computational reasoning during inference.
Upcoming Advancements
- Smaller, optimized versions of DeepSeek R1 for consumer devices.
- Improved reinforcement learning for more adaptive AI behavior.
- Advanced memory retention to allow long-term learning.
Conclusion: DeepSeek R1 is The Future of AI Reasoning
DeepSeek R1 isn’t just another AI it is reshaping the future of reasoning in artificial intelligence. With its CoT reasoning, self-learning mechanisms, and open-source accessibility, it stands as one of the most transparent, intelligent, and forward-thinking AI models available today.